What drives parallel or convergent evolution?
Similar evolutionary changes arising in independently evolving populations, known as parallel or convergent evolution, are often taken to be evidence of strong selection. However, heterogeneity in mutation rate across the genome has the potential to play an equally important role. The relative contribution of these two processes and the potential for other factors to further modify patterns of parallel evolution in natural populations is still unclear and difficult to test. However, replicated experiments evolving populations of microbes have begun to provide some insight into the drivers of parallel evolution. I use a combination of experimental and statistical approaches to explore these important processes, with the ultimate goal of trying to understand if and when evolution is predictable.
Watch my talk on this theme as part of the ASN Young Investigators Prize symposium at the Evolution 2016 meeting.


Using replicated experimental evolution of populations of P. fluorescens, I have characterized parallel evolutionary changes across multiple levels of biological organization from phenotype, to gene, to nucleotide, in multiple environments, and showing that selection environment, and so ecological context, can strongly influence the degree of parallel evolution (Bailey et al 2015).
Future experimental work is aimed at identifying specific factors of the selection environment, such as distance from the fitness optimum, level of pleiotropy, and population size, that previous theoretical models (e.g. Chevin et al 2010) suggest may drive/ impede the probability of parallel evolution. Results from these experiments will be explored and quantified using customized statistical approaches discussed below.
Statistical models quantifying processes driving parallel evolution
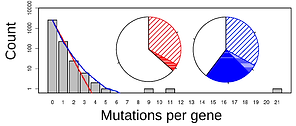
I currently use two approaches to probe experimental evolution sequence data with an aim to characterize and quantify factors driving parallel evolution. The first approach is to fit Poisson regression models incorporating a range of gene-level variables (e.g. level of gene expression, codon bias, recombination rate, GC content, etc.) to sequence data (in the form of counts of mutations per
gene) and identify those variables that can significantly predict which genes tend to bear mutations.
With the second approach, I fit stochastic models assuming an underlying Poisson process to sequence data. Here, the goal is to partition variation in the data into variation arising from heterogeneity in mutation rate and variation arising from heterogeneity in the intesity of selection. This approach allows us to characterize the relative contributions of mutation and selection heterogeneity in driving patterns of parallel evolution. As we begin to apply this model framework across a range of organisms and environmental conditions, we will ope to be able to identify in a quantitative way how the characteristics of a particular systems effect the key processes underlying evolution and so drive differences in the probability of parallel evoltuion from one system to another. This work is an ongoing collaboration with Thomas Bataillon, Johanna Bertl, and François Blanquart.
Interested to see how these models (or something similar) could be applied to your sequence data?
Parallel evolution in experiments with Pseudomonas fluorescens